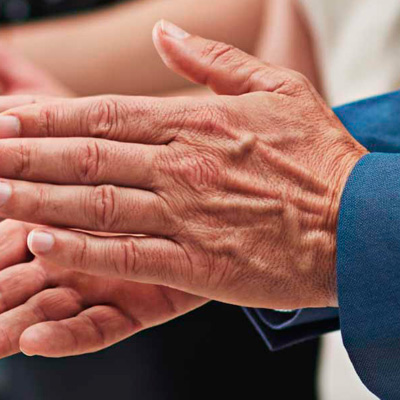
JOSE LUIS AZNARTE MELLADO
CATEDRÁTICO DE UNIVERSIDAD
INTELIGENCIA ARTIFICIAL
ESCUELA TÉCN.SUP INGENIERÍA INFORMÁTICA
(+34) 91398-9688
Academic Information
Computer Science Engineer (University of Granada, 2003)
PhD in Computer Science (University of Granada, 2008)
Teaching
Asignaturas de Master:
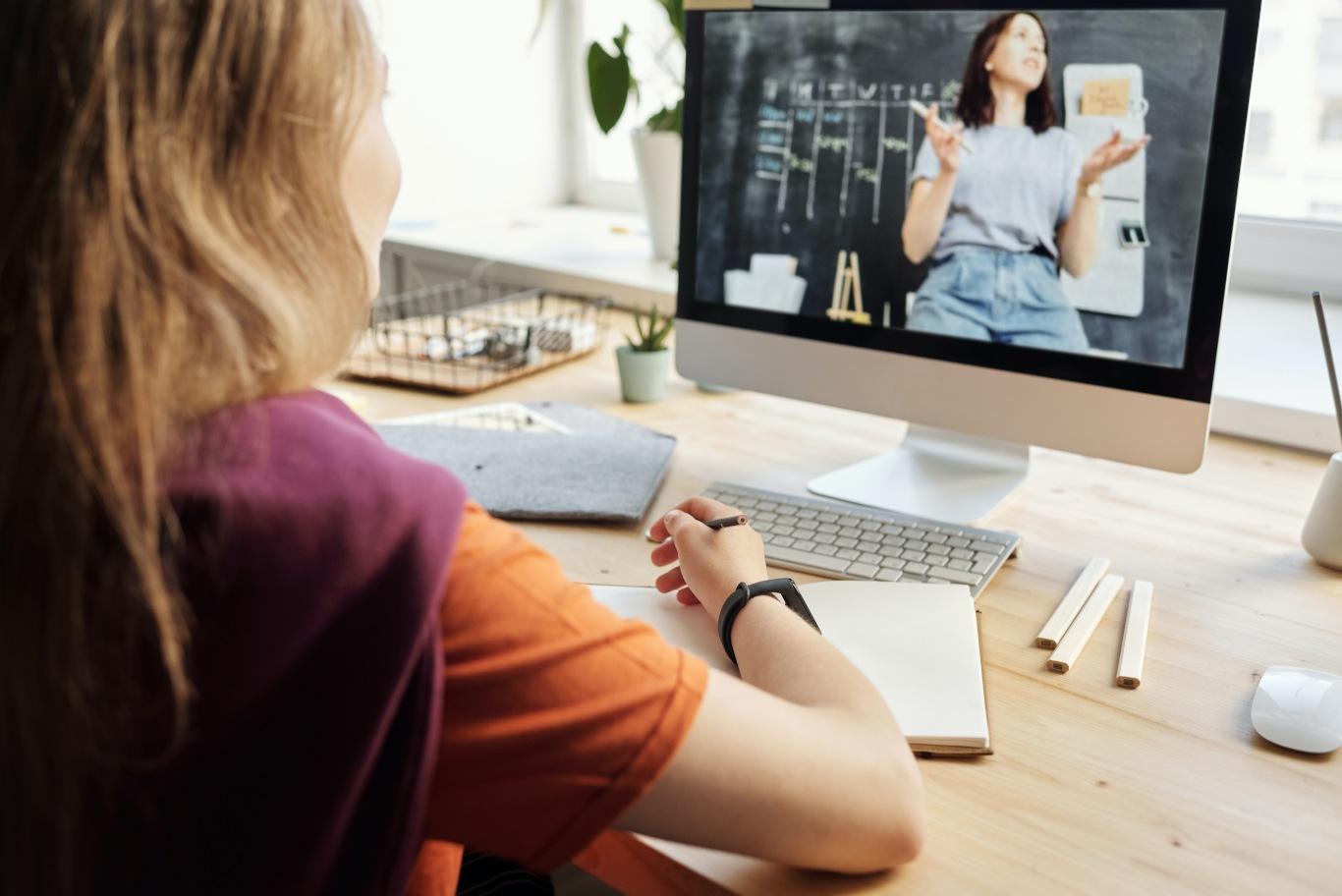
N.º of recognized sections of teacher evaluation
2Research
Research interests
-
Time series forecasting with computational intelligence models
Many scientific fields produce time-ordered data, which are usually not independent from one another. Great benefits (not only economic) can be obtained on these scientific fields by analysing these data, and particularly by properly forecasting them.
Some applications developed during the last years:
- Airborne pollen: from historical records and from meteorological measures and predictions, forecasting the concentrations of pollen of different species.
- Air quality: also from historical records, meteorological measures and predictions and other values as traffic, the idea is to analyse, model and forecast the concentrations of different pollutants in the air.
- Health related series: forecasting the number of hospital admissions from pollution and other variables.
- Earthquakes: from historic earthquake series, the discovery of precursor events helps forecasting the occurrence of potentially hazardous earthquakes.
- Pollen image classification Pollen grains of different species are extremely difficult to distinguish. Through the use of deep learning, we are able to provide pollen identification and classification. This is useful in a variety of disciplines ranging from palynology to forensics.
N.º of recognized sections of research activity
3Publications
-
PUBLICATIONS IN MAGAZINES
42/ V. Sevillano, J.L. Aznarte, Scarce is enough: Image Augmentation to Improve Automatic Pollen Classification in Small Datasets, under review.
41/ R. Gadea, J.L. Aznarte, Attention to traffic forecasting: improving predictions with temporal graph attention networks, under review. Preprint: https://doi.org/10.36227/techrxiv.19732483.v1.
40/ L. Gutiérrez, R. de Medrano, J.L. Aznarte, COVID-19 forecasting with deep learning: a distressing survey. Under review. Preprint: https://doi.org/10.36227/techrxiv.16855189.v1.
39/ K. Sherratt et al., Predictive performance of multi-model ensemble forecasts of COVID-19 across European nations. Under review. Preprint: https://www.medrxiv.org/content/10.1101/2022.06.16.22276024v1.
38/ Olmedo Lucerón C, Díez Domingo J, Expósito Singh D, Moriña Soler D, Aznarte JL, Almagro Pedreño J, Limia Sánchez A. Predicciones de tres modelos matemáticos en relación a la estrategia de vacunación frente a la COVID-19 en España. Junio de 2021. Rev Esp Salud Pública. 2022; 96. https://pubmed.ncbi.nlm.nih.gov/35179148.
37/ J.L. Aznarte, M. Melendo, J.M. Lacruz, Sobre el uso de tecnologías de reconocimiento facial en la universidad: el caso de la UNED, Revista Iberoamericana de Educación a Distancia (2021). DOI: https://doi.org/10.5944/ried.25.1.31533.
36/ R. de Medrano, J.L. Aznarte, A New Spatio-Temporal Neural Network Approach for Traffic Accident Forecasting, Applied Artificial Intelligence (2021). https://doi.org/10.1080/08839514.2021.1935588.
35/ S. Pérez-Vasseur, J.L. Aznarte, Forecasting the full distribution of NO2 concentrations for extreme pollution episodes, Scientific Reports, 11 (2021). https://doi.org/10.1038/s41598-021-90063-3.
34/ R. de Medrano, V. de Buen Remiro, J.L. Aznarte, SOCAIRE: Forecasting and Monitoring Urban Air Quality in Madrid, Environmental Modelling & Software, 143 (2021). https://www.sciencedirect.com/science/article/pii/S1364815221001274.
33/ R. de Medrano, J.L. Aznarte, On the inclusion of spatial information for spatio-temporal neural networks, Neural Computing and Applications, 2021. https://doi.org/10.1007/s00521-021-06111-6.
32/ N. Abellán, B. Jiménez-García, J.L. Aznarte, E. Baquedano, M. Domínguez-Rodrigo, Deep learning classification of tooth scores made by different carnivores: achieving high accuracy when comparing African carnivore taxa and testing the hominin shift in the balance of power, Archaeological and Anthropological Sciences, 13:31 (2021). https://doi.org/10.1007/s12520-021-01273-9
31/ B. Jiménez-García, J.L. Aznarte, N. Abellán, E. Baquedano and M. Domínguez-Rodrigo, Deep learning improves taphonomic resolution: high accuracy in differentiating tooth marks made by lions and jaguars, J. R. Soc. Interface 17: 20200446 (2020). https://dx.doi.org/10.1098/rsif.2020.0446
30/ R. de Medrano, J.L. Aznarte, A Spatio-Temporal Spot-Forecasting Framework for Urban Traffic Prediction, Applied Soft Computing, Volume 96, November 2020. https://doi.org/10.1016/j.asoc.2020.106615. Preprint: https://arxiv.org/abs/2003.13977.
29/ J.L. Aznarte, Consideraciones éticas en torno al uso de tecnologías basadas en datos masivos en la UNED, Revista Iberoamericana de Educación a Distancia (2020). https://revistas.uned.es/index.php/ried/issue/view/1381
28/ V. Sevillano, K. Holt, J.L. Aznarte, Precise automatic classification of 46 different pollen types with convolutional neural networks, PLoS ONE (2020): https://doi.org/10.1371/journal.pone.0229751
27/ R. Navares, J.L Aznarte, Deep learning architecture to predict daily hospital admissions, Neural Computing and Applications. https://doi.org/10.1007/s00521-020-04840-8
26/ R. Navares, J.L. Aznarte, C. Linares, J. Díaz, Direct assessment of health impacts from traffic intensity on hospital admissions in Madrid, Environmental Research, vol. 184, 2020. https://doi.org/10.1016/j.envres.2020.109254
25/ M. Vega, J.L. Aznarte, Explaining pollution predictions: a study on NO2 concentrations, Ecological Informatics, Volume 56, March 2020. https://doi.org/10.1016/j.ecoinf.2019.101039
24/ R. Navares, J.L. Aznarte, Predicting Air Quality with Deep Learning LSTM: Towards Comprehensive Models, Ecological Informatics, 2020, vol. 55: https://doi.org/10.1016/j.ecoinf.2019.101019.
23/ R. Navares, J.L. Aznarte, Geographical Imputation of Missing Poaceae Pollen Data via Convolutional Neural Networks, Atmosphere, 2019, 10(11), 717. https://doi.org/10.3390/atmos10110717
22/ D. Valput, R. Navares, J.L. Aznarte, Forecasting hourly NO2 concentrations in urban areas by ensembling machine learning and mesoscale models: a case study in Madrid, Neural Computing and Applications (2019): https://link.springer.com/article/10.1007/s00521-019-04442-z
21/ R. Navares, J.L. Aznarte, Forecasting Plantago airborne pollen: improving feature selection through clustering and Friedman tests, Theoretical and Applied Climatology (2019): https://link.springer.com/article/10.1007/s00704-019-02954-1
20/ V. Sevillano, J.L. Aznarte, Improving Classification of Pollen Grain Images of the POLEN23E Dataset through three different Applications of Deep Learning Convolutional Neural Networks, PLoS ONE (2018): https://doi.org/10.1371/journal.pone.0201807.
19/ E. Florido-Navarro, G. Asencio-Cortés, J.L. Aznarte, F. Martinez-Alvarez, A novel tree-based algorithm to discover seismic patterns in earthquake catalogs. Computers & Geosciences, Volume 115, June 2018, Pages 96-104: https://doi.org/10.1016/j.cageo.2018.03.005.
18/ R. Navares, J. Díaz, C. Linares, J.L. Aznarte, Comparing ARIMA and computational intelligence methods to forecast daily hospital admissions due to circulatory and respiratory causes in Madrid, Stochastic Environmental Research and Risk Assessment (2018): https://doi.org/10.1007/s00477-018-1519-z.
17/ J.L. Aznarte, Probabilistic forecasting for extreme NO2 pollution episodes, Environmental Pollution, Volume 229, October 2017, Pages 321-328: https://doi.org/10.1016/j.envpol.2017.05.079
16/ R. Navares, J.L. Aznarte, What are the most important variables for airborne pollen forecasting?, Science of the Total Environment (2017), Volume 579.
15/ E. Florido, F. Martínez-Álvarez, A. Morales, J.L. Aznarte, Earthquake prediction based on artificial neural networks: A survey, Croatian Operational Research Review 7 (2), 159-169 (2016).
14/ R. Navares, J.L. Aznarte, Predicting the Poaceae pollen season: six month-ahead forecasting and identification of relevant features. Int J Biometeorol (2016). doi:10.1007/s00484-016-1242-8
13/ J.L. Aznarte, N. Siebert, Dynamic Line Rating Using Numerical Weather Predictions and Machine Learning: a Case Study. IEEE Trans. Power Delivery, Volume: PP, Issue: 99 (2016). doi:10.1109/TPWRD.2016.2543818
12/ E. Florido, F. Martínez-Álvarez, A. Morales-Esteban, J. Reyes, J.L. Aznarte-Mellado, Detecting precursory patterns to enhance earthquake prediction in Chile. Computers & Geosciences, Volume 76, March 2015, Pages 112-120.
11/ C. Bergmeir, I. Triguero Velázquez, D. Molina, J.L. Aznarte, J.M. Benítez, Time Series Modeling and Forecasting Using Memetic Algorithms for Regime-switching Models. IEEE Transactions on Neural Networks and Learning Systems, vol. 23, no. 11, pp. 1841–1847, 2012.
10/ J.L. Aznarte, J. Alcalá-Fdez, A. Arauzo-Azofra, J.M. Benítez, Financial time series forecasting with a bio-inspired fuzzy model. Expert Systems with Applications, vol. 39 (2012) 12302-12309.
9/ J.L. Aznarte, J.M. Benítez, Testing for heteroskedasticity of the residuals in fuzzy rule-based models. Applied Intelligence Vol. 34, Issue: 3 (2011), 386-394 (doi:10.1007/s10489-011-0288-x). Paper selected for publication in a special number of the journal dedicated to the conference IEA/AIE 2010.
8/ A. Arauzo-Azofra, J. L. Aznarte M., J. M. Benítez, Empirical Study of Feature Selection Methods Based on Individual Feature Evaluation for Classification Problems. Expert Systems with Applications Vol. 38, Issue: 7 (2011), 8170-8177.
7/ J.L. Aznarte, J. Alcalá-Fdez, A. Arauzo-Azofra, J.M. Benítez, Fuzzy autoregressive rules: towards linguistic time series forecasting. Econometric Reviews Vol. 30, Issue 6 (2011) 646-668.
6/ J.L. Aznarte, J.M. Benítez, Neural-autoregressive time series models with fuzzy equivalences. IEEE Transactions on Neural Networks Vol. 21, No. 9 (2010), 1434-1445.
5/ J.L. Aznarte, M.C. Medeiros, J.M. Benítez, Testing for linear independence of the residuals in fuzzy rule-based models. International Journal of Uncertainty, Fuzziness and Knowledge-Based Systems, Vol. 18, No. 4 (2010) 371–387. Paper selected for publication in a special number of the journal dedicated to the IX ISDA Conference (Pisa 2009).
4/ J.L. Aznarte, M.C. Medeiros, J.M. Benítez, Linearity testing against a fuzzy rule-based model. Fuzzy Sets and Systems 161 (2010) 1836-1851, doi:10.1016/j.fss.2010.01.005.
3/ J.L. Aznarte, J.M. Benítez, J.L. Castro, Smooth Transition Autoregressive Models and Fuzzy Rule-based Systems: Functional Equivalence and Consequences. Fuzzy Sets and Systems 158 (2007) 2734-2745, doi:10.1016/j.fss.2007.03.021.
2/ J.L. Aznarte, R. Navajas, C. Rubio, M. Ruiz, M. A. Garrido, SatDNA Analyzer: a computing tool for satellite-DNA evolutionary Analysis. Bioinformatics 23:6 (2007) 767-768, doi:10.1093/bioinformatics/btm005.
1/ J.L. Aznarte, D. Nieto Lugilde, J.M. Benítez, F. Alba Sánchez, C. de Linares Fernández, Forecasting airborne pollen concentration time series with neural and neuro-fuzzy models. Expert Systems with Applications 32 (2007) 1218-1225, doi:10.1016/j.eswa.2006.02.011.